The 7 Biggest Mistakes Companies are Making in AI and Agent Adoption (and How to Overcome Them)
- Nufar Gaspar
- Apr 22
- 3 min read
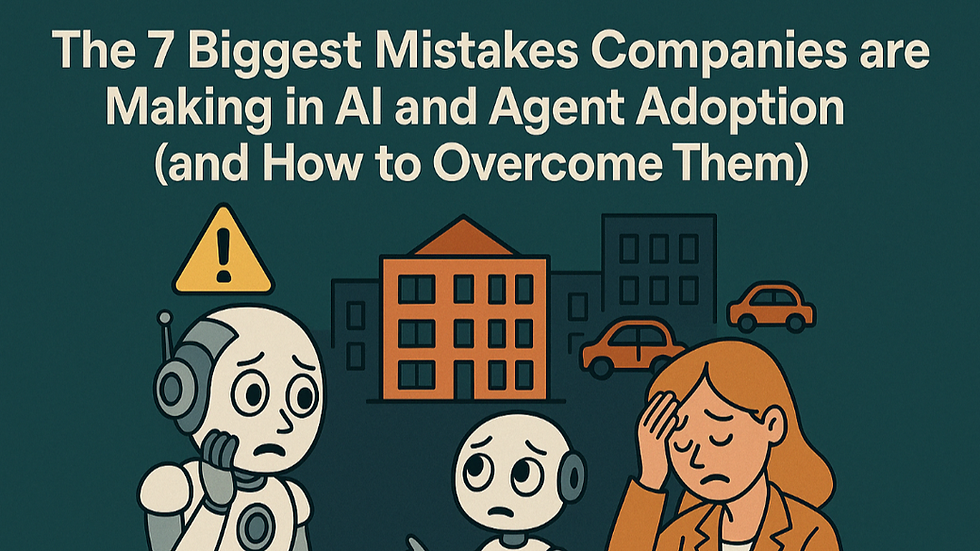
AI has been massively hyped over the past few years, with exponential technological growth seemingly outpacing practical adoption. Despite significant investment in AI initiatives, many companies are struggling to achieve the expected transformational impact. In my recent months working closely with companies across various sizes and industries, I've identified seven critical mistakes companies make in their AI and agent adoption journeys. Below, I'll share these common pitfalls and, more importantly, practical advice on how to avoid or overcome them.
1. Single-direction approach to AI innovation
Companies often mistakenly rely solely on either grassroots innovation or a top-down approach:
Grassroots efforts alone typically stall at the demo or pilot stage because employees lack the bandwidth to scale these ideas.
Top-down directives without employee engagement lead to resistance or lack of practical adoption. For instance, in several audited companies, CEOs envisioned AI-driven transformation while employees were concerned about job security.
💡What you should do:
Combine top-down guidance with grassroots innovation.
Involve employees early and consistently to align innovation with practical execution and alleviate fears.
Provide funding and recognition for successful bottom-up initiatives.
2. Haphazard AI adoption
When companies let “everyone” handle AI without clear accountability, it leads to chaotic adoption, redundant efforts, and poor results. Often, no clear policy exists, and employees remain unsure about responsibilities and expectations.
💡What you should do:
Clearly assign responsibility for AI adoption.
Use a balanced approach of incentives (carrots) and clear guidelines (mini-sticks).
Establish dedicated AI teams or councils to streamline efforts and foster focused innovation.
3. Unrealistic expectations for AI outcomes
Media hype can lead companies to believe AI is simple to implement. This results in unrealistic expectations:
Leaders expect quick transitions from demos to production, overlooking substantial scaling and support efforts.
Users expect perfect performance from AI, failing to account for the technology’s current limitations.
Companies underestimate the complexity of developing high-quality, enterprise-grade AI agents.
Employees expect immediate value without investing the necessary time to learn and adapt to new AI tools.
💡What you should do:
Set realistic expectations based on AI capabilities today.
Plan for and allocate appropriate resources for AI development and adoption.
Begin with straightforward, proven use cases to build momentum and expand from there.
4. Poor data management and access
Effective AI and agent deployments depend heavily on quality data. Unfortunately, data in most companies is scattered, duplicated, or insufficiently organized, significantly delaying AI implementation.
💡What you should do:
Prioritize building a robust Retrieval-Augmented Generation (RAG) foundation or similar systems to integrate and access data sources.
Allocate dedicated time and resources to clean and structure your data.
If you're starting fresh, design your data systems with future AI and agent integration in mind.
5. Poor vendor and tool selection processes
Common pitfalls in tool selection include:
Unnecessary in-house builds, wasting time and resources.
Blindly trusting major vendors without adequate vetting.
Endless piloting without actionable outcomes.
Overly restrictive or overly permissive policies on tool usage.
💡What you should do:
Clearly define criteria for build-buy-hire decisions for each use case.
Carefully vet vendors based on track record and relevance to your specific needs.
Set clear policies on adopting and piloting new tools, ensuring efficient decision-making processes.
Involve relevant stakeholders in vendor evaluations.
6. Siloed approaches and poor communication
Employees frequently lack awareness about available AI tools or capabilities within their own organizations. This occurs even in smaller companies, highlighting poor internal communication.
💡What you should do:
Create internal networks of AI champions to promote sharing and collaboration.
Develop clear communication channels or platforms to inform everyone about available AI resources and company policies.
Publicly recognize contributions and innovations to encourage widespread adoption.
7. Delaying AI agent adoption due to immature technology
Waiting until AI technology matures further might seem prudent but can cost companies critical competitive advantages and learning opportunities.
💡What you should do:
Adopt a proactive yet realistic strategy.
Start immediately with practical, achievable use cases.
Collaborate with proven platforms or vendors to accelerate adoption and learning.
Continuously measure results and refine your approach.
To successfully navigate these common pitfalls and accelerate your AI adoption journey, consider reaching out for personalized guidance through my consultation services. Together, we can ensure your company not only avoids these mistakes but thrives by strategically leveraging AI and agents.
Want to dive deeper? Listen to my full conversation about these challenges on the AI Daily Brief podcast here.
Comments